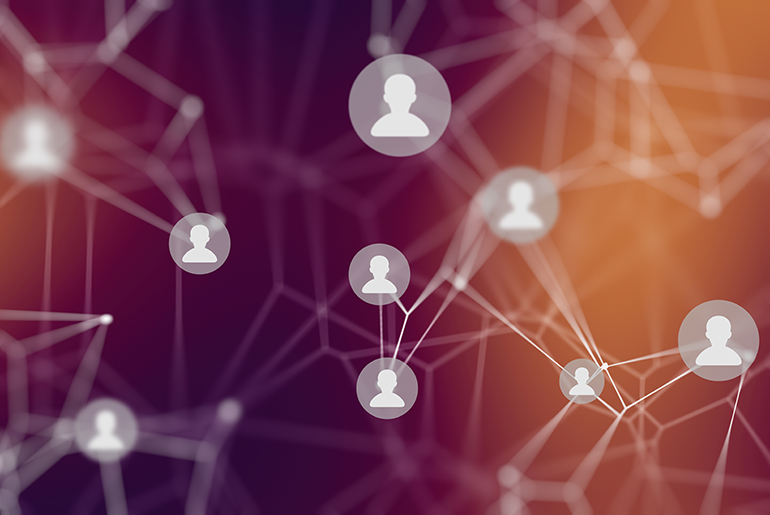
Networks have been widely used to represent real-world information systems such as social media networks or a healthcare provider network. Traditional network data often operates without human input, until now. In a traditional data-driven network-embedding framework, only network analytics algorithms are considered. They are purely data driven, meaning that the algorithms do not take into consideration any human prior knowledge that may be helpful in many situations.
Human prior knowledge means that there are some pieces of information that we as humans might know and understand that a computer may not automatically determine. Dr. Xia (Ben) Hu, assistant professor in the Department of Computer Science and Engineering at Texas A&M University, was awarded the National Science Foundation (NSF) Faculty Early Career Development Award (CAREER) to build a human-centric network-embedding framework, in which human prior knowledge would be properly modeled and integrated in the framework process in contrast to the traditional data-driven network-embedding framework.
Prior to conducting network analysis, Hu operated on the assumption that some people are more influential than others on social networks. Individuals, such as national leaders and movie stars, are much more influential than many others on Twitter because of their leadership, popularity and activity. Without including that human prior knowledge into the network, the computer might not pick up on that.
Many types of human prior knowledge are dynamic and can change from day to day. For example, a current president’s influence on social media will be more important after he is elected versus before. Hu said that all of these factors should be considered and be tackled while modeling the network embedding problem and understanding the network.
Hu proposes to systematically investigate three types of human knowledge from the node-, edge- and community-level in a network, and to integrate them into a combined framework. An example of the edge-level human knowledge is the connections between contacts on a social network – where some connections between contacts are stronger than others on social networks. While the traditional data driven framework may not accurately indicate one’s connections with others, Hu’s project will integrate human prior knowledge that will accurately show those connections on social networks.
“For example, I may be closer with my students than another professional colleague from another university,” Hu said. “However, on social networks it appears that I may or may not have those connections with others.”
In healthcare, there are many types of human prior knowledge that can be beneficial to include in the framework so that diagnoses and medical history can be more accurate in patients seeking medical care.
“In healthcare systems there are many other types of human knowledge,” Hu said. “For example, we know some people are (related). That’s why we should take family disease history into consideration in disease prediction for AI driving healthcare analytics.”
Not only will this project impact social networks and healthcare, but it will also impact the critical infrastructure by implementing human prior knowledge of the connections between power stations into the network embedding problem.
“Before hurricanes and natural disasters it is critical to understand which power stations are more important than others by conducting these network analytics,” Hu said. “Some power stations are more important because if they are ever damaged, the whole area power systems will be down.”
If researchers are able to analyze which power stations are the most critical to keep stable, it can help prevent unnecessary outages during natural disasters.
This research enables data analysts and domain experts, such as doctors, to handle network data from real-world information systems with abundant human knowledge for scientific discovery, which was extremely difficult, if not impossible, before.
“While existing studies focused on data-driven approaches, this project is to investigate a novel direction to explore how human knowledge could enhance network embedding and how the results could be better understood by human beings,” Hu said. “The successful outcome of this research will lead to advances in providing an effective embedding, which is essential in analyzing real-world networks with human knowledge.”
Hu also plans to make sure that data science education can be received by a larger audience in order to make a broader impact. His plan is to not only make an impact through classroom teaching, but to make data science education publicly available and try to make sure more people receive an education in terms of data science and network analytics by posting them online in different formats.