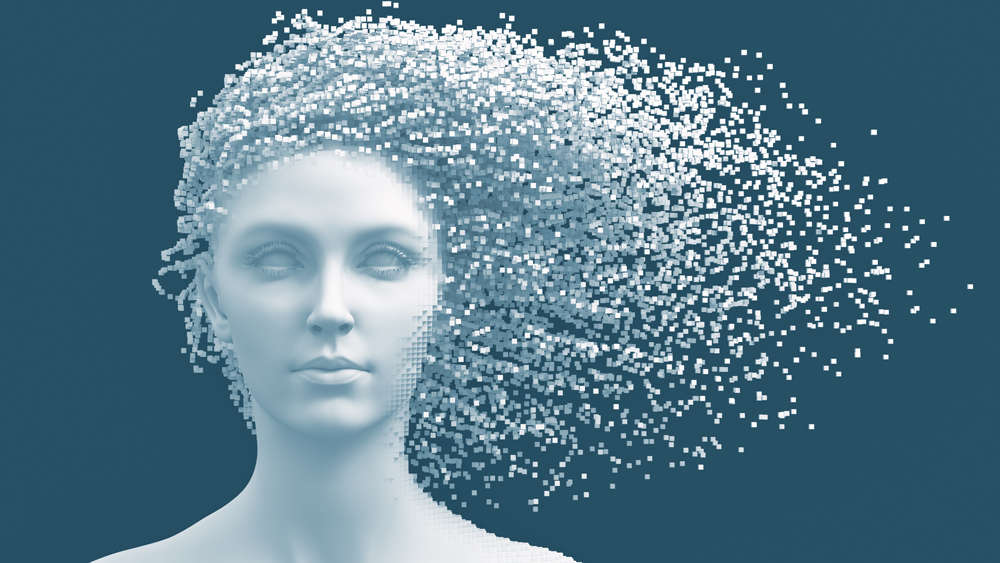
Deepfake images of people look real. They pose in realistic settings and, in the case of videos, can emote almost naturally. However, everything about deepfakes is synthetic – just a series of codes that come together to form an image of a person who doesn’t exist.
However, as Dr. Freddie Witherden, assistant professor in the Department of Ocean Engineering at Texas A&M University, discussed in a paper titled Fourier Spectrum Discrepancies in Deep Network Generated Images , the images and bots behind the falsified faces are not without fault.
Used largely as bots on social media platforms to spread fake news and sway opinion, deepfakes are a global and interdisciplinary issue that begs the question: Is seeing really believing?
“It seems, initially, like something vastly different to what an ocean engineer would normally do day to day. But quite a bit of my day to day research involves machine learning, applications of machine learning and, in the same way that deepfakes try and synthesize realistic looking pictures of people, some of my research involves using the same technology to simulate fluid flows or generating fluid flows without having to do a full simulation,” Witherden said.
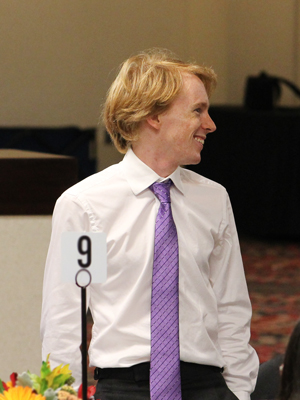
His research began when ocean engineering doctoral student Tarik Dzanic approached him with a desire to test ways to differentiate deepfake images from real ones. Together, they dove into the intricate details of pictures – comparing and contrasting how each scaled and differed.
“Our approach is a little bit different from what we've seen in the literature,” Witherden said. “The idea is that if you look at a real photo, which is of a person, of an object, that will typically be taken with a camera, and so that photo will have some degree of noise associated with it. If you zoom in and look at the pixels of a high-resolution photograph, there's typically some kind of noise pattern.”
Because that noise does not bother people viewing an image, attention is normally not paid to it. Similarly, he explained, the algorithms that are trained to generate deepfake images also do not pay attention to the noise, either. Rather, deepfake algorithms focus on the big picture and recognizable features of a face, such as a mouth, nose and eyes.
And that was the key for Witherden and Dzanic.
“They're not concerned about the minutia associated with the noise and so they don't make any effort into trying to get that noise profile correct,” Witherden said. “And so deepfake images that you see online at the moment generally have a different noise profile to those of real images that were captured with the camera. And, so, by doing some very simple signal processing, we can identify these discrepancies and thus discriminate between real images or fake images.”
Hear more about how Witherden and Dzanic are exposing deepfakes in a recent podcast interview. Listen on your favorite audio platform, or stream Witherden’s podcast episode directly from the SoundBytes website.