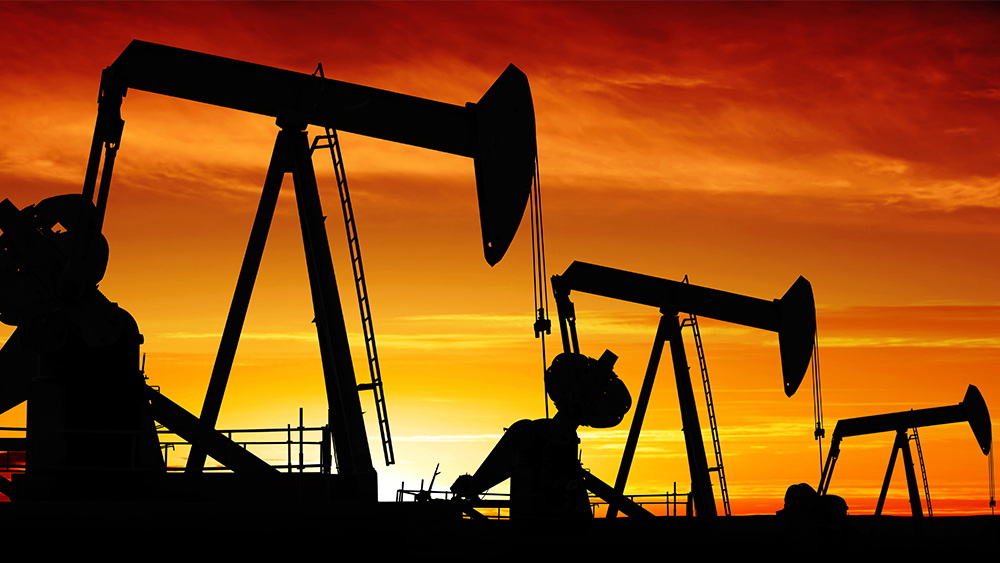
Petroleum engineering graduate student Eliza Ganguly recently received a Society of Petrophysicists and Well Log Analysts Foundation grant for her research in petrophysics – the study of the physical and chemical properties of rocks and rock-fluid interaction.
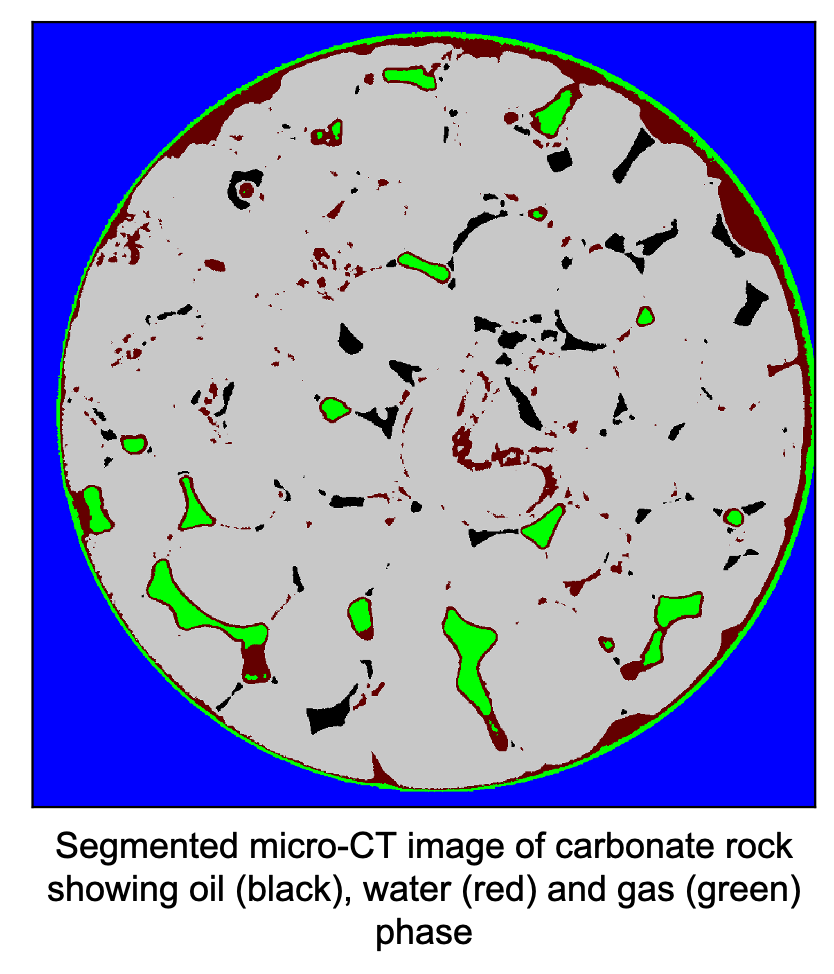
This area of study focuses on how pores in the subsurface are interconnected and how these connections control the storage, transport and recovery of hydrocarbon.
Ganguly’s research, supervised by her advisor, Dr. Siddarth Misra, specifically focuses on quantifying fluid phase connectivity from whole-core images of a carbonate sample, after various fluid injection scenarios. She has created a machine learning-based workflow that uses the segmentation of the microscopic images of the rock by dividing them into distinct components, followed by computation of fluid phase connectivity.
The developed metrics can standardize and speed up the measurement of connectivity to facilitate the characterization of properties and processes of the rock material.
“In petroleum engineering, one of the most important parameters governing the production of oil is porosity, which quantifies the pore space present in a sample, whereas connectivity is an important petrophysical property that directly controls the recovery of fluid from pore space,” Ganguly said.
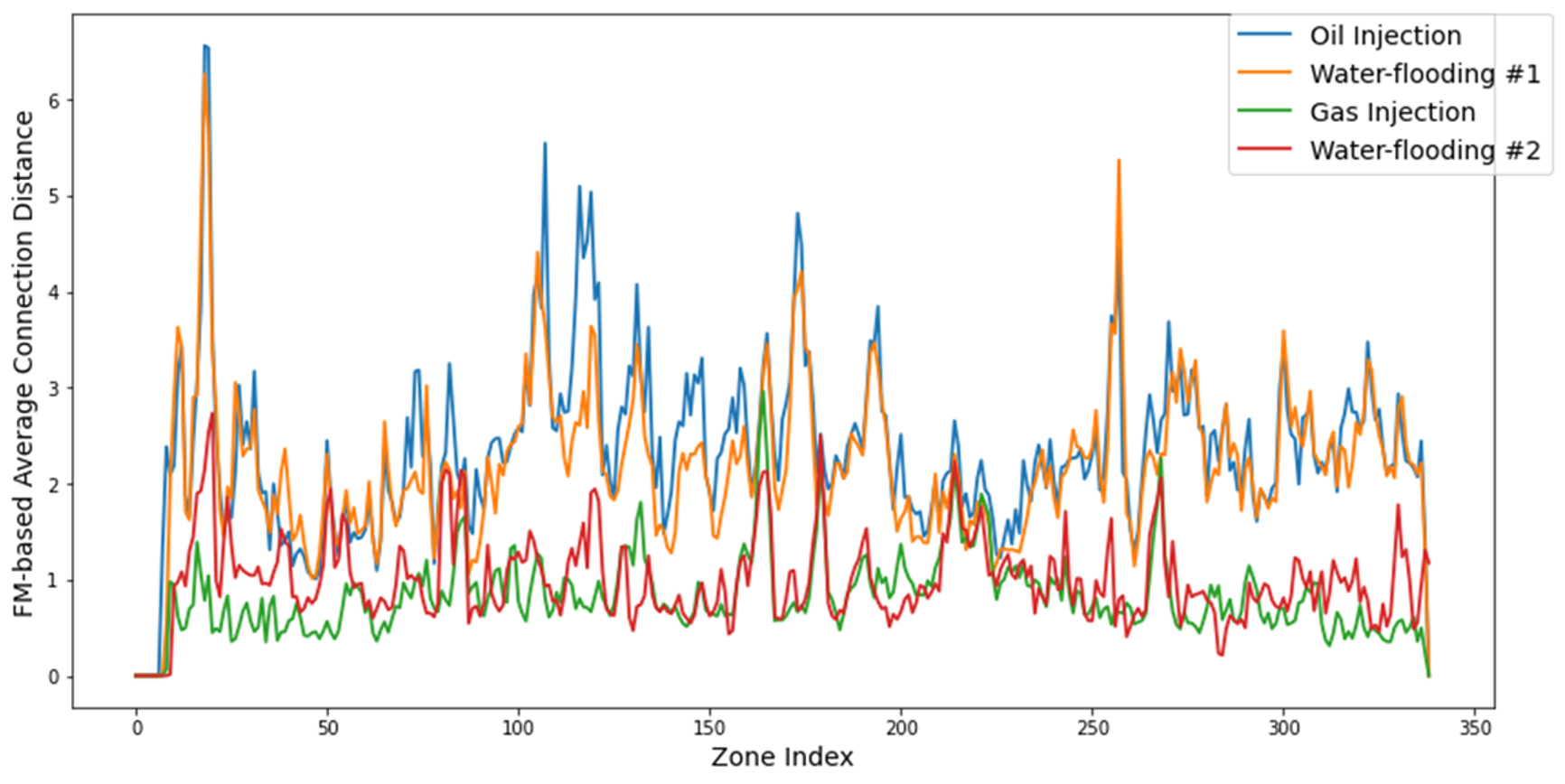
In order to “see” how the fluid components are connected, the workflow uses multiple microscopic images, maps or scans of the rock sample to create a three-dimensional volume. By breaking the component up into multiple parts, it is easier to see each component. The connectivity metrics are then applied to obtain the connectivity values for oil, water and gas components. The results from the metrics help identify the parts in the rock where there is maximum fluid recovery.
“We quantify many things in our day-to-day lives,” Ganguly said. “From age, height, temperature and speed, comparing the different parameters of each gives us valuable insights and helps us make decisions like, avoid speeding tickets.”
With the funds from the grant, Ganguly will make her machine learning-based workflow accessible to petrophysicists across the world. By using her workflows and testing them for accuracy, they will be improved and lead to a better understanding of connectivity.