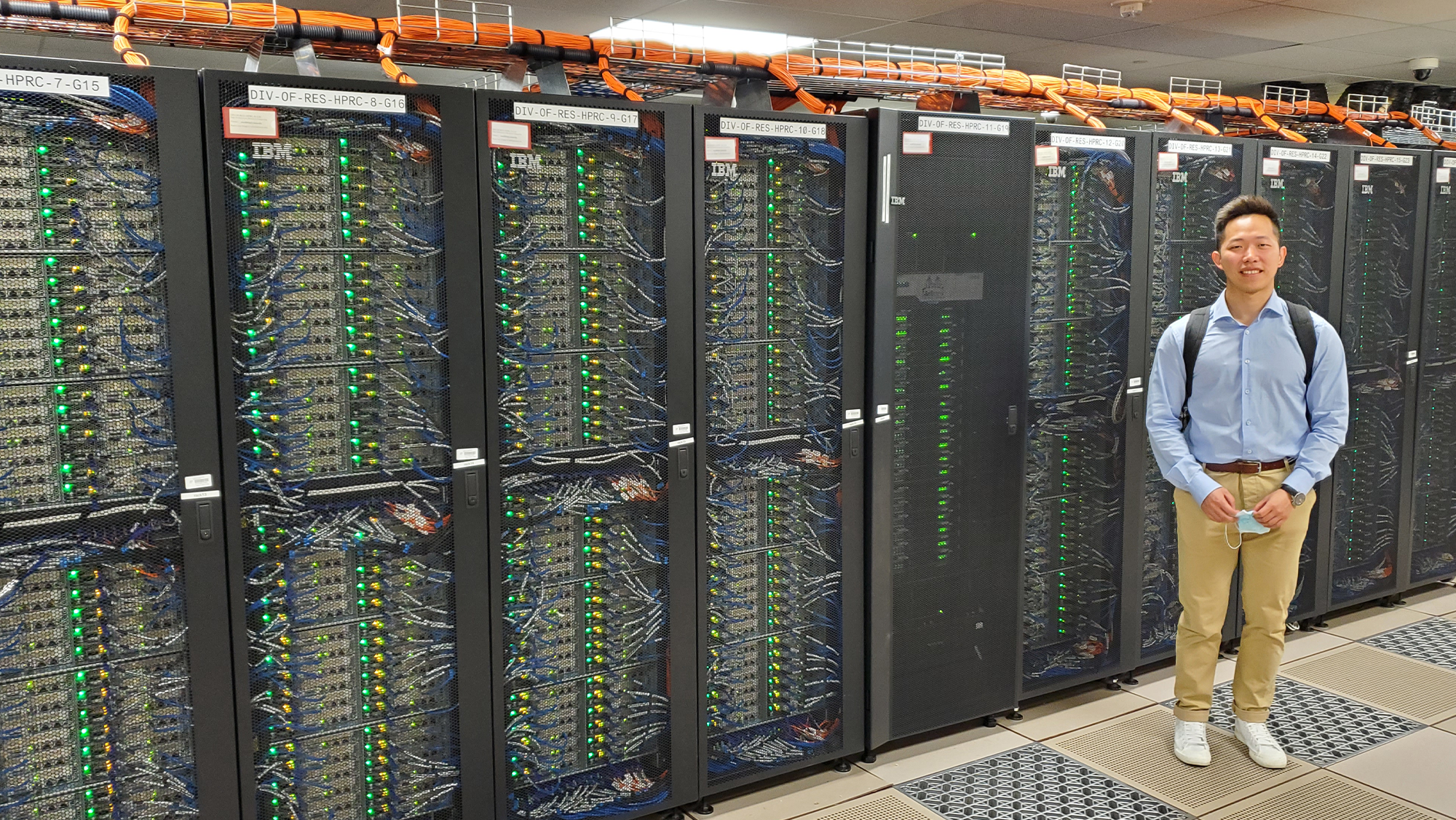
Jiayi Lin used a super computer to solve optimization problems. These computers are housed in Texas A&M’s High Performance Research Computer facility. |
Image: Courtesy of Jiayi Lin
Researchers at Texas A&M University and George Mason University are investigating the utilization of population-level data in an effort to codify quarantine policies for policymakers. This will ultimately alleviate the spread of diseases during epidemics and pandemics from multiple angles, including mass screening, quarantining and vaccine distribution.
Dr. Hrayer Aprahamian, assistant professor, and Jiayi Lin, doctoral student, in the Wm Michael Barnes ’64 Department of Industrial and Systems Engineering at Texas A&M, and Dr. Hadi El-Amine, assistant professor of systems engineering and operations research at George Mason University, have identified quarantine policies using subject-risk information to mitigate the spread of disease while also recognizing the potential for the negative economic impact.
“In order to identify the best possible policy we formulate this decision problem within an optimization framework and use a range of tools to be able to solve the resulting problem accurately and efficiently,” Aprahamian said. “Doing so enables us to solve the problem for realistic problem instances.”
Their research aims to not only provide practitioners, administrators and policymakers with evidence-based insights and recommendations on mitigating the spread of disease in pandemics, but to also demonstrate that operations research and mathematical tools can be used to successfully divulge more optimized mitigation policies to effectively combat the spread of diseases.
“This paper provides an efficient solution scheme to a class of challenging optimization problems that arise in numerous real-world applications, like crew scheduling, vehicle routing, inventory management, group testing and bin packing,” Lin said. “From a practical standpoint, this paper addresses an important question that many practitioners have continued to struggle with, how can one use the vast amount of COVID-19 data to shape informed data-driven policies?”
The researchers attempt to answer this question by providing a mathematical framework to identify quarantine policies that are effective in mitigating the spread of disease while considering both subject-specific risk information and overall economic impact. This distinction arises from the fact that there is no one-size-fits-all policy.
The team conducted a COVID-19 case study using real-world risk data for the state of Minnesota — which was achieved by simulating a realistic community based on census data — and then ran an optimization model on this particular community. The resulting models were solved using Texas A&M’s High Performance Research Computing facilities. To achieve comprehensive results, the experiment was repeated for a range of realistic parameter values. To measure the benefits of the proposed policies, the team compared their solution to more conventional one-dimensional policies.
“Targeted policies tailored to the specific needs of the local population are recommended. Such specific solutions, however, are often complex and require us to work closely with local leaders in order to successfully implement them,” Aprahamian said. “This research demonstrates that the identified data-driven policies outperform conventional measures by both reducing the spread of the disease and having less economic impact.”
“One observation that is worth highlighting is that the results reveal that taking no action at all is never the best solution for a wide range of realistic parameter values, even in the most extreme of cases,” Lin said.
“These results, when scaled, translate to hundreds of thousands of fewer infections and millions of dollars of savings,” Aprahamian said. “Such high-level insights are of great value as they can be used by larger national or worldwide agencies to urge local administrators to take action, especially at the early stages of the pandemic.”
Dr. Hrayer Aprahamian, assistant professor, and Jiayi Lin, doctoral student, in the Wm Michael Barnes ’64 Department of Industrial and Systems Engineering at Texas A&M, and Dr. Hadi El-Amine, assistant professor of systems engineering and operations research at George Mason University, have identified quarantine policies using subject-risk information to mitigate the spread of disease while also recognizing the potential for the negative economic impact.
“In order to identify the best possible policy we formulate this decision problem within an optimization framework and use a range of tools to be able to solve the resulting problem accurately and efficiently,” Aprahamian said. “Doing so enables us to solve the problem for realistic problem instances.”
Their research aims to not only provide practitioners, administrators and policymakers with evidence-based insights and recommendations on mitigating the spread of disease in pandemics, but to also demonstrate that operations research and mathematical tools can be used to successfully divulge more optimized mitigation policies to effectively combat the spread of diseases.
“This paper provides an efficient solution scheme to a class of challenging optimization problems that arise in numerous real-world applications, like crew scheduling, vehicle routing, inventory management, group testing and bin packing,” Lin said. “From a practical standpoint, this paper addresses an important question that many practitioners have continued to struggle with, how can one use the vast amount of COVID-19 data to shape informed data-driven policies?”
The researchers attempt to answer this question by providing a mathematical framework to identify quarantine policies that are effective in mitigating the spread of disease while considering both subject-specific risk information and overall economic impact. This distinction arises from the fact that there is no one-size-fits-all policy.
The team conducted a COVID-19 case study using real-world risk data for the state of Minnesota — which was achieved by simulating a realistic community based on census data — and then ran an optimization model on this particular community. The resulting models were solved using Texas A&M’s High Performance Research Computing facilities. To achieve comprehensive results, the experiment was repeated for a range of realistic parameter values. To measure the benefits of the proposed policies, the team compared their solution to more conventional one-dimensional policies.
“Targeted policies tailored to the specific needs of the local population are recommended. Such specific solutions, however, are often complex and require us to work closely with local leaders in order to successfully implement them,” Aprahamian said. “This research demonstrates that the identified data-driven policies outperform conventional measures by both reducing the spread of the disease and having less economic impact.”
“One observation that is worth highlighting is that the results reveal that taking no action at all is never the best solution for a wide range of realistic parameter values, even in the most extreme of cases,” Lin said.
“These results, when scaled, translate to hundreds of thousands of fewer infections and millions of dollars of savings,” Aprahamian said. “Such high-level insights are of great value as they can be used by larger national or worldwide agencies to urge local administrators to take action, especially at the early stages of the pandemic.”