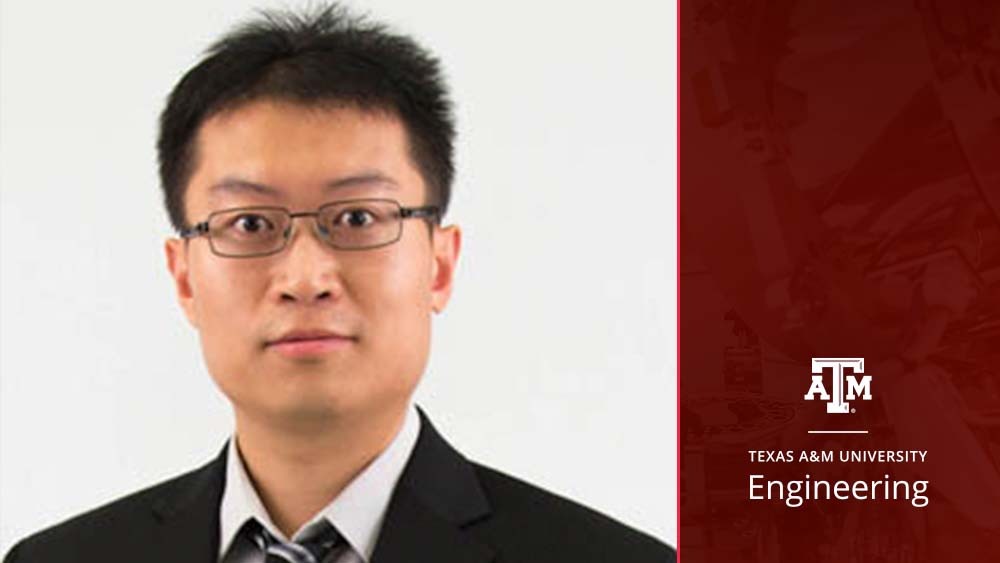
Advances in directional drilling and the control of drilling robotics could be critical for discovering potential microbial life and signs of water resources on Mars.
On Earth, such technology is equally impactful — significantly decreasing the cost of producing geothermal renewable energy, reducing the environmental impact of constructing new clean energy sites and accessing underground oil reserves, and increasing production safety. Additionally, it allows scientists at the South Pole to evaluate climate change patterns and predict future impacts, such as the evolution of global warming.
The challenge lies in controlling this technology. Between overcoming barriers in the environment, such as rocky terrain, vibrations and wellbore formation interaction located deep underground, and the risk of human error, the operation of directional drilling provides a unique set of operating conditions.
Dr. Xingyong Song, associate professor in the Department of Engineering Technology and Industrial Distribution at Texas A&M University, received a National Science Foundation (NSF) Faculty Early Career Development Program (CAREER) grant to advance the control of deep underground directional drilling systems. Through this project, he aims to create a more reliable drilling process of automating controls by developing a new computational framework for operating directional drilling technology in this world and beyond.
As Song explained, automation and machine intelligence have advanced dramatically in recent years but have lagged behind in its implementation in the drilling industry. In its stead, many processes still rely heavily on human workers and their experience, response time and ability to react.
This is an issue when dealing with stimuli and feedback at the end of a drill bit that is 10,000 feet away and underground.
“In drilling, you’re allowing sensors to see what’s underground and when this information is transmitted to the surface a lot of times there is a time delay,” Song said. “And if you only rely on humans to make decisions and send back a control command after this feedback comes in, oftentimes it will already be too late.”
Additionally, regardless how experienced a worker is, with as complicated as a drilling environment is, having one or two people have full decision-making power makes it an unreliable strategy.
So, Song asks, why not hand the reigns over to machine-learning techniques, based on big data and advanced modeling of the whole directional drilling process to allow computers to make the decisions for the field engineers?
Over the next five years, Song plans to utilize the $638,000 awarded to him to develop a new directional drilling control model and a new computational algorithm to address state-barrier avoidance control that will address the complex barriers faced by the drilling industry. This will be used to teach artificial intelligence programs to handle the complex obstacles it may face when handling real-world directional drilling applications.