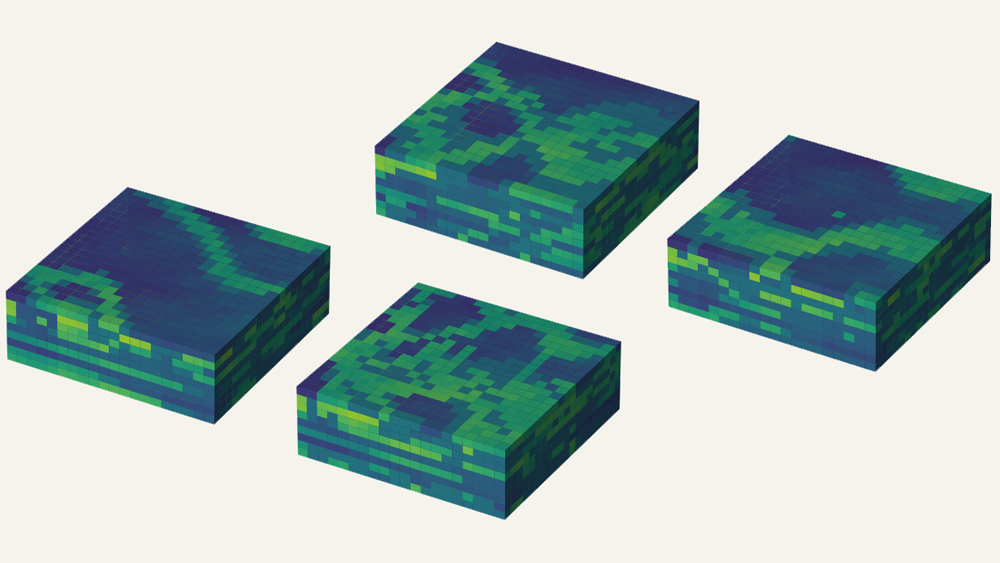
Two primary challenges that petroleum engineers face are finding oil in the Earth’s subsurface and making it flow to enable production. The subsurface contains a huge variety of materials and is underground and out of sight, so the process is like finding a needle in a large haystack in the dark. Over the past few years, oil and gas companies have deployed advanced sensor arrays in well sites to gather the information needed to shed light on these issues. However, the amount of data collected is too large to be handled by standard methods of effective analysis.
For the past several months, graduate student researchers Matteo Caponi, Polina Churilova and Yusuf Falola at Texas A&M University have been using machine learning, where computers are trained how to learn by programmers, to effectively pare down the amount of data the sensors are producing. The students were then able to teach modeling software the algorithms they created — algorithms that knew what to look for, what to ignore and how to depict the answers needed by companies to make informed decisions.
“It is very exciting to work with real data and understand how different techniques can come together to predict reservoir properties,” said Caponi.
The two ongoing projects are funded by upstream oil and gas companies ConocoPhillips and Berry Petroleum, and both will evaluate the results in August for future applications in the field. The students’ work was overseen by Dr. Siddharth Misra, the Ted H. Smith, Jr. ’75 and Max R. Vordenbaum ‘73 DVG Associate Professor in the Harold Vance Department of Petroleum Engineering at Texas A&M. The projects are industry and academic partnerships engaged by the applied research group, Data-Driven Intelligent Characterization and Engineering (DICE), led by Misra. He created DICE to encourage oil and gas companies to use data, machine learning and analytics to lower operation costs, better assess risks and improve resource recovery.
“Today’s sensing technology is easy to deploy, relatively cheap and stores a lot of data, which leads to these big dataset sizes,” said Misra. “But you cannot decisively work with such big data unless you extract the most relevant and useful information.”
Sensors can take a broad range of measurements, from soundwave reflections to pressure changes to temperature fluctuations and more, but most of this information isn’t needed to solve the problems of finding and recovering subsurface oil. Without some way of reducing the data down to the core values required to model oil production pathways, feedback from the sensors remains overwhelming and useless.
Research like this improves the understanding and control of subsurface earth resources, which could reduce the cost and carbon footprint of producing oil.
Misra said that machine learning is the best way to make sense of massive data because the created algorithms can be trained to rapidly sift the information according to the structure or parameters needed to solve specific problems. Spatial data in the form of images, seismic volumes and geological models work best in the training process, he says.
One challenge the students faced was the amount of time it took to train their algorithms, which had to become capable of teaching themselves to find the right ‘needles’ in the reservoirs. Misra introduced the students to the most successful methods of robust feature extraction and deep learning, which use neural network creation. The students created the networks by treating each small step of data reduction as a simplified task the algorithms had to learn. The algorithms’ neural networks grew level by level, with each level representing a different task the algorithms mastered.
“I learned so much in just a few months,” said Falola. “These techniques can greatly reduce reservoir model running times, improve production forecasts, accurately predict formation water production and more.”
As the work progressed and the students gained confidence, they approached one of the biggest hurdles in this advanced work: overcoming the tendency of data-driven models to overfit the data. Overfit occurs when an algorithm mistakenly believes that a subsurface noise or data stream is important when it really isn’t.
The students applied three methods to convince the algorithms to ignore the extra noise and find the correct answer. First, they used artificial, simplified well-site data where the oil location and flow paths were already known and easily discovered. Then, to see if the algorithms could distinguish the correct answer from the wrong ones, they used loss training where the data contained a few possibilities for bad predictions. Finally, to teach the algorithms more about the varieties of positive predictions the software should focus on, the students introduced them to initial feature-extraction data from wells that were already producing oil.
Once the algorithms could handle the basics, they were tested on data that was either complex but incomplete or complex and full of unnecessary information. When successfully prepared, the algorithms could apply their knowledge to any type of similar data packets to uncover relevant answers.
“I am fascinated to work at the intersection of deep learning and petroleum engineering,” said Churilova. “I think the technologies that we develop have the potential to revolutionize the industry.”
The students frequently interacted with engineers and geoscientists working with ConocoPhillips and Berry Petroleum to find out what oil production problems the machine-learning algorithms needed to tackle. This valuable interaction is why Misra created the research group DICE — to engage his students in experience opportunities with industry. However, that isn’t his only goal.
“Research like this improves the understanding and control of subsurface earth resources, which could reduce the cost and carbon footprint of producing oil,” said Misra. “It also improves industry confidence to reach out to academic institutions for solutions to their problems.”