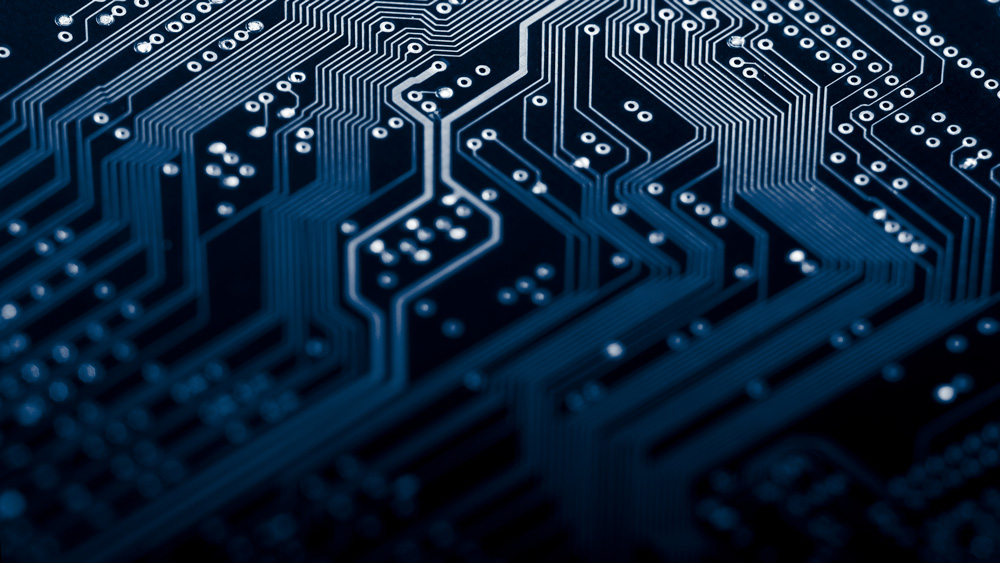
Guiding a drill bit through the earth with the same cutting-edge automation used for steering airplanes and cars is currently impossible because dense subsurface materials slow the speed of any transmitted instructions down to a crawl.
Two recent Texas A&M University engineering graduates are bypassing this slowdown with an advanced smart tool that processes sensor data, renders subsurface models, maps a route and steers the drilling — all while sitting down hole behind the drill bit.
Drs. Enrique Losoya ’22 and Narendra Vishnumolakala ’22 had the idea for the device while they were graduate students. Together with another student, Connor Ust, they founded their own company, Teale Engineering LLC, in 2020 to take on the challenge of creating their product. In 2021, Teale received a phase I grant of $256,000 nondilutive seed capital from the National Science Foundation’s (NSF) Small Business Technology Program.
“The objective of phase I was to perform research, prove the technical feasibility and build the proof-of-concept hardware and software needed,” said Losoya.
The former students enlisted Dr. Eduardo Gildin, L.F. Peterson ’36 Professor in the Harold Vance Department of Petroleum Engineering; Sheelabhadra Dey, a doctoral student in the Department of Computer Science and Engineering; and former student Paul Deere ’92, an accomplished innovator and expert in downhole and measurement-while-drilling technology, as the research team for the project.
Currently, drillers steer drill bits using a bottom hole assembly that contains a bias or bend. The drill string — the pipe attached to the assembly — is pushed down on from above and constantly rotated to make the bit go straight down. If the drill string is not rotated, the bit will drill in the direction of the bias. Drillers know which way a bit is heading by following a tracker in the assembly. They direct the bit by reading reservoir models or maps generated by computers using data from subsurface sensors. Unfortunately, the sensor data is transmitted up through thousands of feet of rock and other materials to reach those computers. While modern commercial cellular transmissions can travel up to 10,000,000,000 bits, or 10 Gigabits, per second through air or space, the Earth’s subsurface slows those speeds down to a stunning 2 to 6 bits per second.
“Drilling using models is somewhat like blindly driving a car in the dark with only the instructions on the dashboard screen to guide you,” said Gildin, who serves as the team’s technical advisor. “Slow transmission speeds mean those instructions take a while to produce.”
The team is putting the tool downhole to be as close as possible to the sensors. That way, it can process the data and model a map in near real time. Putting the device right behind the drill bit also means it can steer with faster reaction times.
Several obstacles stood in the way of their product. First, the team had to build a physical device small enough to fit in the space behind a drill bit yet big enough to hold all the hardware. Second, they had to produce the software needed for processing and rendering the sensor data. Third, they had to create animated simulations to train their tool’s machine-learning algorithms how to understand its unique view of the reservoir and model — as a car driver would if looking through a 360-degree windshield — and how to manipulate the drill string behind it. Plus, the tool had to look at the reservoir model the way a driller would, with production needs and safety issues as the top destinations and priorities.
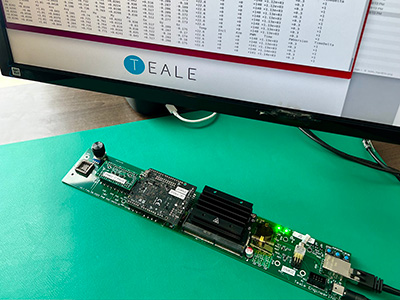
For the first prototype, the former students borrowed what they could from off-the-shelf hardware while creating a lot of software. Gildin and Dey helped develop the reinforcement-learning algorithms needed for the tool to understand how to correctly judge the best drilling course and bit speeds from the models. Losoya and Vishnumolakala created the virtual environment and real-time linearized model simulations necessary for testing the learning algorithms’ abilities. The work was done on Teale’s servers and Texas A&M supercomputers under Gildin’s guidance.
Dey said the custom drilling simulators were developed using popular simulation engines like the Unity Physics Engine, a “mature 3D development platform typically used for video games.”
Several months of trial and error eventually led to successful lab tests, but the work is far from over. Now that the simulations and algorithms work, the team must replace all the off-the-shelf technology with more robust equipment that can handle the harsh conditions downhole.
“We are seeking a phase II grant from the NSF,” said Losoya. “That’s where we scale, refine and focus on developing a field-ready intelligent prototype and product.”
“Costs are higher as we experiment with new configurations and materials,” said Deere, the team’s executive and commercialization advisor. “We will work with the large downhole tool providers we know to scale our production so we can provide a smart and affordable product for operators. I am excited to help commercialize this research and expect it will greatly impact the directional drilling market.”
If the team can produce an economical product smart enough to follow the best drilling routes every time, the tool could make drilling oil and geothermal wells more accurate, more profitable and far safer.
Losoya and Vishnumolakala both graduated with master’s degrees in petroleum engineering and doctoral degrees in multidisciplinary engineering from Texas A&M. Gildin, who mentored them during their time in petroleum engineering, pointed out how rare this opportunity is.
“This is a tangible project where a product is developed,” Gildin said. “It’s a side of research few students get to see because most research is scholarly.”
Because this project has strong research components, Losoya, Vishnumolakala, Dey and Gildin are producing a paper on the phase I work that is slated for publication in 2023.