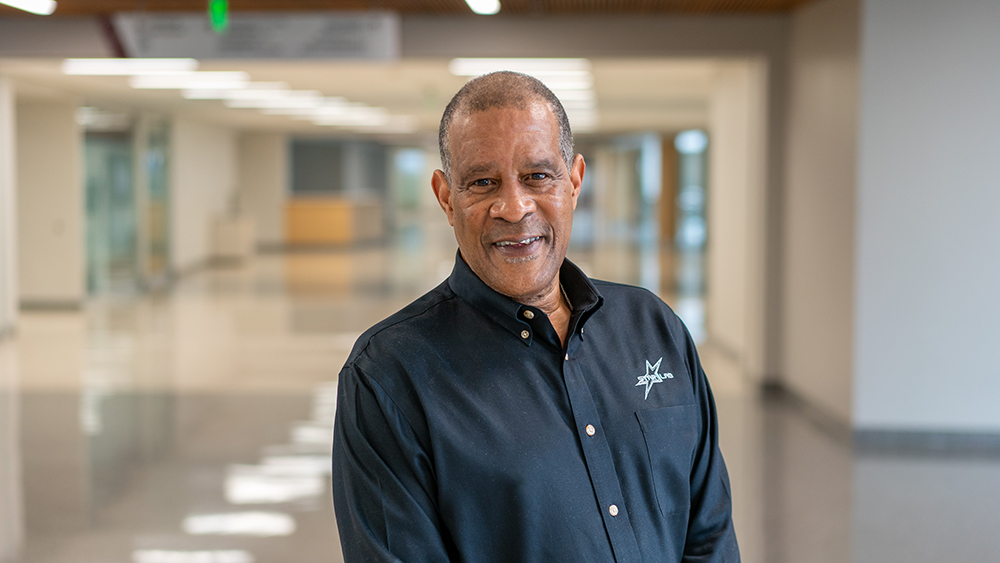
In the future, when airplanes are more autonomous, what if having a few sensors in a pilot’s cap allowed for human-machine teaming (when a human and a machine work together)? As long as things run normally, no human interaction with the airplane would be needed. But if something goes wrong, the airplane will need to know if the pilot is aware of it, and if they are not, the sensors could let the plane know if the pilot is paying attention.
Texas A&M University Distinguished Professor Dr. James E. Hubbard set out to develop a model to estimate human decision-making to improve human-machine teaming in the future for people who work in industrial fields, manufacturing processes and space. With 50 years of engineering experience, a background in adaptive structures and a growing interest in human cognition, Hubbard did just that.
“The focus of this research is to get measurements from a human non-invasively that could be interpreted by a machine so that when it's running autonomously, it understands if you're paying attention or not,” said Dr. Tristan Griffith, Hubbard’s graduate student.
Using a mathematical modeling technique for identifying earthquakes as a guide for output-only system identification, Hubbard and Griffith discovered that electroencephalogram (EEG), or brainwave data, is the most accessible way to obtain output from the brain. Using well-understood tools of estimation and control theory, they set out to identify a model in terms of canonical engineering dynamics to see how the human-machine team changed in real time.
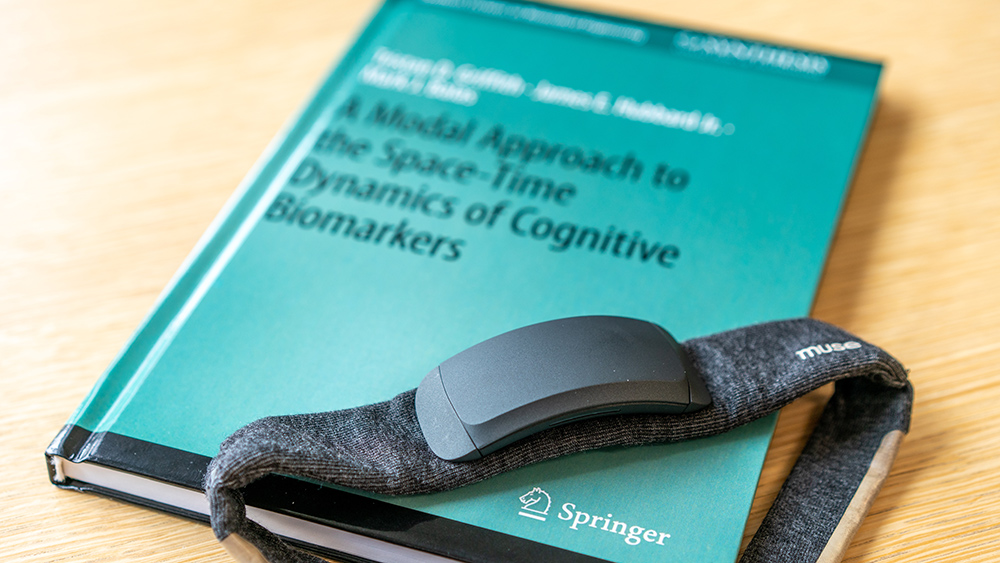
“The question we're trying to answer is, ‘How does the machine keep track of a human's supervisory process?’ Because in the future, people aren't going to be driving cars, they're going to be supervising the car driving itself,” Griffith said. “It would be really great if you could measure how somebody was paying attention, and then alert them if they weren't.”
Research in this area has been virtually untouched until Hubbard, Griffith and Dr. Mark J. Balas, world-renowned expert in adaptive systems, wrote a book on it, “A Model Approach to the Space-Time Dynamics of Cognitive Biomarkers.”
In an increasingly automated world where cars drive themselves and robots build machinery, the flow of information between people and machines is at risk of disruption, called mode conflict. As a result, human-machine symbiosis is needed to enhance efficiency and protect individuals in positions, such as astronauts and pilots, where interacting with machines is already necessary.
“That’s a challenge for a lot of reasons,” said Griffith. “It's hard to get machines to talk to people like people, and it's hard to get people to talk to machines like machines. There are robust mathematical frameworks for establishing safety criteria and performance criteria for machines, but not for people.”
The researchers created a new model to measure, identify and fingerprint brain waves in real time. They used machine learning, or artificial intelligence (AI), to train their model on a few EEG signal data files from a public database of more than 1,000 data files. Next, they mixed up the files and gave the AI the brain-wave patterns their model put out.
“It ended up being able to identify the person that the brain waves belonged to 100% of the time,” Hubbard said. “In other words, it never failed. We reached in the box, picked out a person’s model, asked who it was, and it accurately identified the person. I was shocked to discover that each person's brain wave is like a fingerprint.”
The next hurdle was to find out how to reduce the probability of error between the output from the EEG channel and the output from the model. Hubbard reached out to Balas, who was able to implement a mathematical correction technique.
“This technique updates the model if the person’s mind drifts,” Hubbard said. “So then, when we looked at the real signal and the new model, there was less than 1% error. They were almost identical.”
In addition, by measuring brain waves at different locations across the brain, they found that people have distinct spatial wave patterns that extend in three dimensions across the brain.
“You can deconstruct the overall activity into a series of 3D spatial patterns and then add up how much of each of the individual patterns in each frequency band equal the whole activity, and it tells you things about human activity, such as engagement and distraction,” Griffith said.
The findings of this project led Hubbard and others to apply the technique to data from a headband. The headband has five sensors. “From that, we can estimate the other channels and still give you a high-resolution image of your brain-wave patterns,” Hubbard said. “Nobody's ever done that using just a headband.”
“The National Institutes of Health just put out a call to fund noninvasive brain-wave imaging,” Hubbard said. “This is going to be a big deal one day. Human-machine symbiosis is possible.”