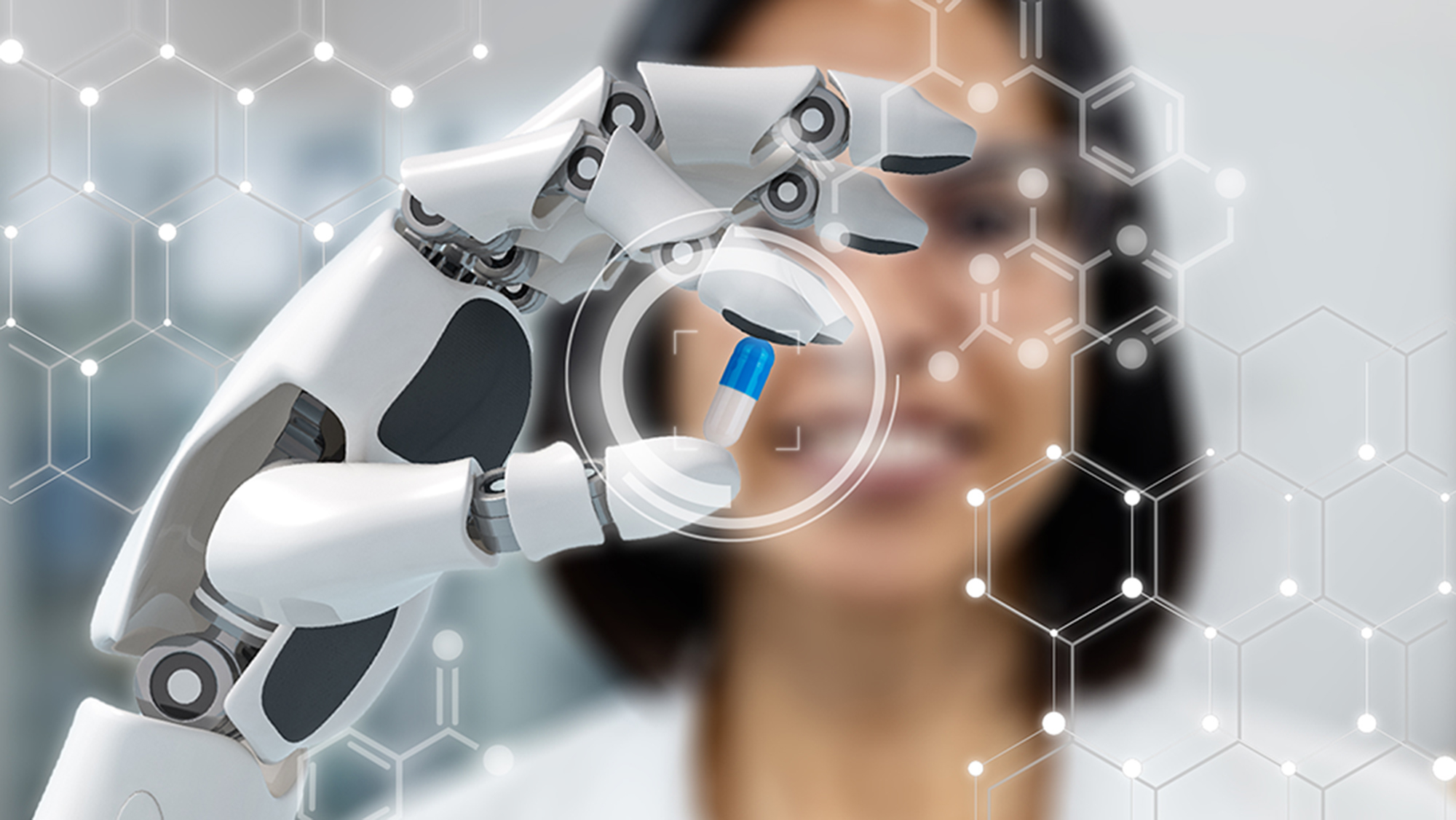
Dr. Joseph Sang-Il Kwon has developed a crystallization simulator named CrystalGPT that impacts the manufacturing process for drugs.
CrystalGPT is the first model that combines well-established physics models and reactor models with a next-gen AI model for a novel ‘best-of-both-worlds’ approach, according to Kwon, a chemical engineering associate professor in Texas A&M University’s Artie McFerrin Department of Chemical Engineering.
When he first started using GPTs, Kwon noticed that despite their popularity, there were hardly any applications in the chemical engineering space. “This realization triggered a month-long brainstorming session that resulted in different ways to build-upon existing transformer models for chemical engineering application,” Kwon said. “My group is one of the few groups in the chemical engineering space that has expertise in this field.”
CrystalGPT and the hybrid crystallization model that integrates with CrystalGPT allow high-accuracy prediction of key process variables in the industrial crystallization process, Kwon said.
The current industrial process is not highly adaptive to various process disturbances or changes to product specifications. As a superior AI-based platform, CrystalGPT will have a huge impact on manufacturing better drugs for the American public.
The goal is not drug discovery, Kwon explained, but to focus on the manufacturing process of already discovered drugs. He hopes to design a manufacturing process that is cost-effective and allows precise control of drug properties.
“Since CrystalGPT can function as a highly accurate and speedy prediction tool, it can highly expedite the process of simulating a manufacturing process,” Kwon said. “All of these activities result in heavy cost-savings for the drug development process. The exact numbers will vary with company and drug type.”
Since CrystalGPT can function as a highly accurate and speedy prediction tool, it can highly expedite the process of simulating a manufacturing process.
Kwon said that improved drug manufacturing will mean cheaper production costs while still adhering to the high standards for quality set by the U.S. Food and Drug Administration.
“Given that currently in the U.S. there is a general concern for the increasing cost of life-saving and basic over-the-counter drugs, increased scale and efficiency in the drug manufacturing process will help in reducing the costs and increasing the quality of these drugs,” Kwon said.
A key factor for better drugs is precise control of the specifications, such as crystal size and shape. These specifications can be improved using CrystalGPT by looking at the dominance of the efficacy, side effects, and potency of the drug.
CrystalGPT has shown enhanced system-to-system transferability across crystal systems and has been demonstrated for high-precision model prediction, along with being integrated with established chemical engineering principles to form AI-enabled hybrid models for implementation in the industry.
“CrystalGPT is the first such model that modifies the large language models to include a ‘time’ component to predict complex reactor dynamics,” Kwon said. “The inclusion of temporal dynamics within a transformer model has been performed by only a handful of researchers in this field, and this will be a pivotal development going forward.”
Because CrystalGPT is fully data-driven, Kwon said that to make it suitable for pilot testing and implementation it was combined with reactor models for crystallization. Kwon then developed an AI-enabled hybrid model that can estimate the hidden process kinetics of any given crystal system.
“This research is also highly relevant to the federal government and US DOE’s recent initiatives on AI,” Kwon said.
Its implementation could increase the economic competitiveness of U.S. industries and boost the welfare of the public, according to Kwon.
“From an advanced manufacturing perspective, CrystalGPT and its hybrid modeling sibling allow better prediction and control of industrial crystallization, thereby establishing a lead over other countries regarding the development of more efficient and precision-heavy processes,” Kwon said.