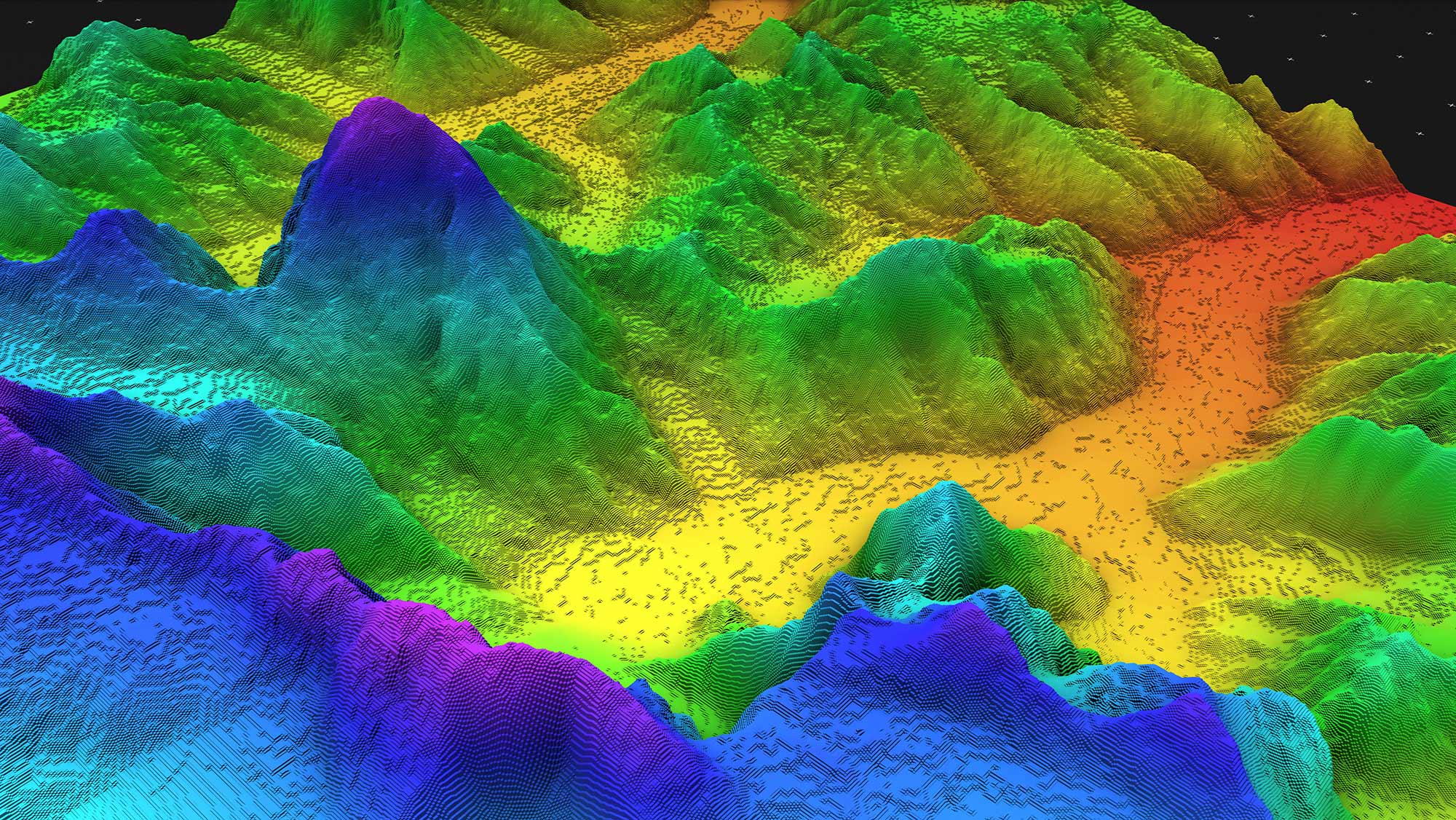
A major challenge for petroleum engineers and geophysicists is a lack of visibility below the Earth’s surface in real time. In order to get a clear picture of what is below the surface, petroleum engineers and geophysicists need to implement a variety of tools and techniques. These methods can be time consuming and costly and provide complex models requiring a trained eye to analyze. Researchers at Texas A&M University have refined and automated this process to shine a light on the once dark subsurface.
Dr. Siddharth Misra, Ted H. Smith, Jr. ’75 and Max R. Vordenbaum ’73 DVG Associate Professor in the Harold Vance Department of Petroleum Engineering, has partnered with ConocoPhillips to develop a more efficient method to map below Earth’s surface. Misra and his team have developed generative AI technology to create more affordable and realistic geomodels.
Q: What is geomodeling?
Misra: A geomodel is a large-scale digital 3D representation of the subsurface earth, depicting various possible scenarios along with their associated probabilities about the subsurface earth. We are never certain of what lies beneath our feet.
These 3D geomodels inherently contain significant uncertainties due to the variability and lack of detailed information about subsurface properties, such as the presence of seals, permeable paths, and faults. Given these uncertainties, it is crucial to quantify the probabilistic nature of the subsurface. This approach enhances the reliability of geomodels, ultimately improving the engineering and sustainability of subsurface earth-resource management.
Geomodeling allows us to understand subsurface earth by obtaining a 3D, high-resolution x-ray of our planet several miles beneath our feet, layer by layer. This process reveals hidden underground features, such as oil and gas reserves, freshwater aquifers, geothermal heat sources, and subsurface volumes for gas and hydrogen storage as well as carbon dioxide sequestration.
Geomodelers play a fascinating role as Earth detectives, tasked with piecing together a picture of the subsurface earth to map the hidden world underground. Unlike detectives who rely on fingerprints and witness accounts, geomodelers gather clues from various subsurface measurements. By combining these diverse pieces of information, geomodelers construct sophisticated 3D models that extend far beyond what we can see directly. These models paint a detailed picture of the unseen world, revealing the presence of valuable resources, fluid pathways, potential leaks, micro-seismic triggers, storage capacity, and heat capacity, to name a few.
Q: Why is Geomodeling necessary?
Misra: Geomodeling is commonly used for managing natural resources, identifying natural hazards, and quantifying geological processes. Geomodels are commonly used to better characterize and plan the development of oil and gas fields, groundwater aquifers, geothermal resources, carbon storage aquifers, and ore deposits, to name a few.
Q: What challenges do you face with geomodeling?
Misra: Normally, mapping the Earth beneath our feet involves massive geomodels with millions of cells. This is like creating a super high-resolution picture, which takes a lot of time and computing power to build and use.
Here's the challenge: after we build these detailed models, we need to adjust them based on real-world observations. This process, called history matching, requires a lot of computing power because the models are so big.
Q: What advancements is your research making in the field of geomodeling?
Misra: Our newly developed workflow tackles this challenge by using a new technique called vector-quantized variational autoencoder, originally developed by Google Deepmind.
Imagine this as a super-efficient data compressor. It shrinks these giant 3D models down to a more manageable size, making them much faster to work with for history matching and other tasks.
But there's another benefit! Once the geomodel is compressed, we used another AI technique, a neural-network-based autoregression-technique called the PixelSNAIL, developed by UC Berkley, to process the above-mentioned low-dimensional representation, to rapidly generate large, realistic 3D geomodels. The two-stage neural-network processing, first compression followed by generation, helps create full, detailed 3D geomodels very quickly. This is like having a high-quality image stored in a tiny file — you can easily get the full picture back whenever you need it.
This is a big leap forward in creating detailed pictures of the hidden world beneath our feet, and it could have a major impact on fields like energy exploration, environmental protection, and understanding our planet's history.
Q: Why is it important for the public to know about this research?
Misra: Overall, this research is a game-changer for geomodeling because it allows scientists to build complex geomodels in a fraction of the time, which can lead to faster discoveries of valuable resources underground. By significantly reducing the computational burden, this technique can save both time and money.
The compressed format makes history matching and other adjustments much faster. Faster modeling allows for exploring a wider range of scenarios, leading to more accurate predictions.
This is a significant leap forward in creating detailed pictures of the hidden world beneath our feet. It could have a major impact on fields like energy exploration, environmental protection, and understanding our planet's history.
Q: Who made this research possible?
Misra: This work was supported by ConocoPhillips through the Data-Driven Intelligent Engineering and Characterization (DICE) research group, which specializes in using sensor data, machine learning and analytics to solve technical challenges related to petroleum engineering, subsurface engineering, and geophysics. DICE Research Group helps industry partners with commercial applications of data-driven engineering, characterization, and modeling of subsurface earth resources for all aspects of upstream exploration, development, and production.
This project was funded by ConocoPhillips, whose personnel also played critical role in this study. Chung-Kan Huang guided us with geomodel processing, while Jose F. Delgado helped provide access to geomodels. Gaoming Li, Gustavo Gomez and John Hand from Conocophillips initiated and supported the project.
Texas A&M graduate students Polina Churilova, Yusuf Falola, and Jungang Chen were a huge part of the project’s success.