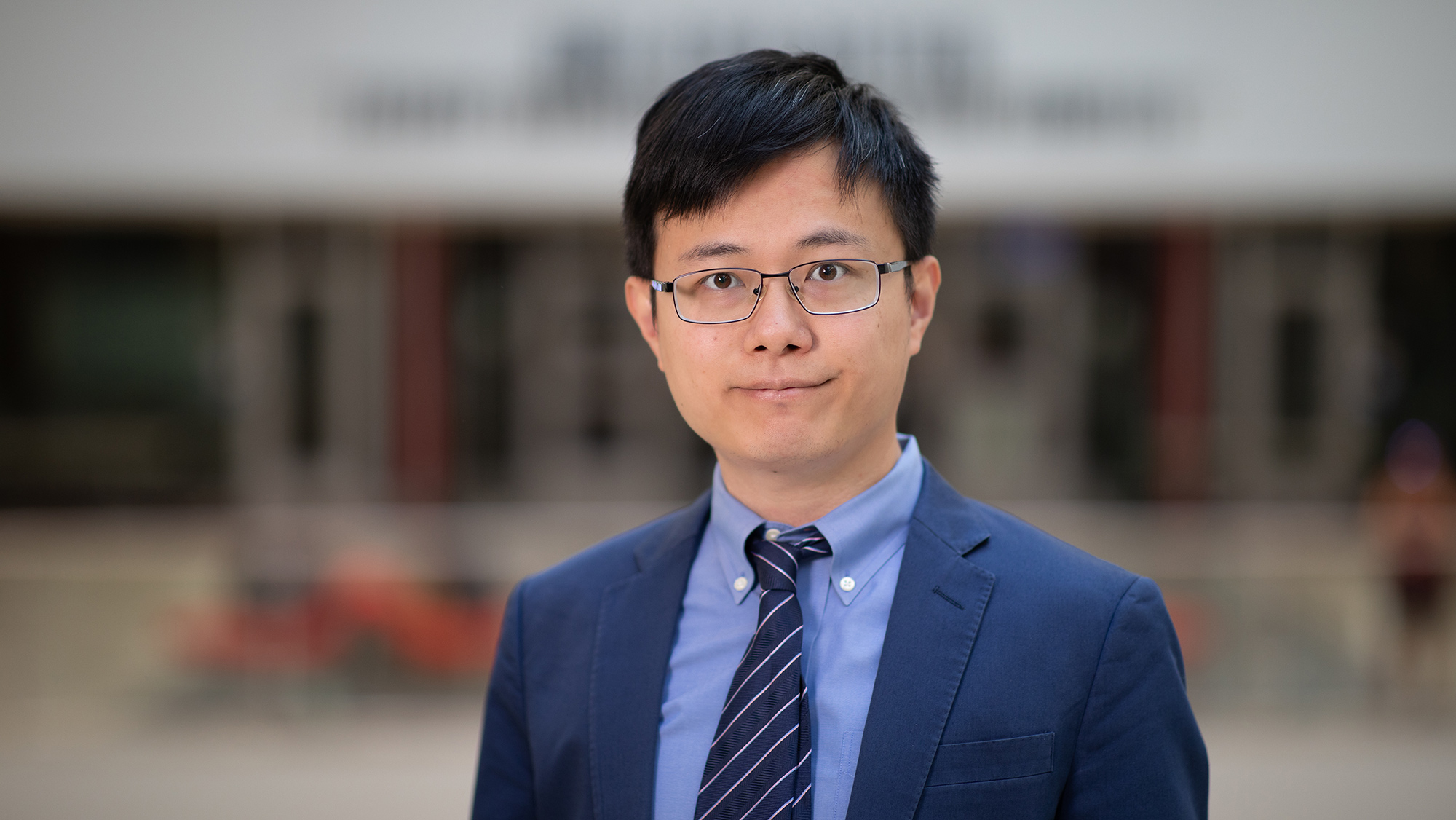
As in many areas of modern life, big data has become a big deal in nuclear engineering. A Texas A&M nuclear engineering professor will investigate artificial intelligence’s ability to harness data to improve the next generation of nuclear reactors.
Yang Liu, an assistant professor in the Texas A&M Department of Nuclear Engineering, is one of four researchers chosen for this year’s Distinguished Early Career Award from the U.S. Department of Energy’s Nuclear Energy University Program. This annual award provides funding for outstanding university faculty early in their careers who will help advance nuclear energy research.
Liu’s research focuses on the role of artificial intelligence (AI) and machine learning (ML) in designing and controlling nuclear reactors. At Texas A&M, he established the Scientific Machine Learning for Advanced Reactor Technologies (SMART) laboratory, where he and his students focus on using ML to enhance simulations, engineering decisions, and the operation of nuclear systems.
During his doctoral studies at North Carolina State University, Liu used ML to research how fluids behave in a nuclear reactor. While a postdoctoral researcher at the University of Michigan, he complemented his computational expertise with more hands-on experimental thermal hydraulics.
“This project is a natural extension of my previous research experience and interests,” Liu said. “We’re trying to bring some innovation into a very traditional, but very important, area: reactor thermal hydraulics, which deals with the safety of a reactor system.”
While some popular AI applications, like ChatGPT, focus on generating text through natural language processing, the role of AI and ML in nuclear engineering is quite different. Here, AI processes and learns vast amount of data from simulations and experiments, enabling researchers to refine their understanding and enhance the design and safety of nuclear reactors.
This project is a natural extension of my previous research experience and interests. We’re trying to bring some innovation into a very traditional, but very important, area: reactor thermal hydraulics, which deals with the safety of a reactor system.
“We are in the era of big data, like on the internet, where people are generating texts, images, and videos on the level of terabytes every day,” Liu said. “In the nuclear engineering community, we're also entering an era of big data, which come from high-fidelity simulations and high-resolution experiments.”
With this award, Liu plans to use ML to simulate and study molten salt reactors, a type of nuclear reactor that originated in the mid-20th century but has seen a resurgence in research interest. These advanced reactors use molten salt to cool down the system and keep it safe. This differs from the commonly used light water reactors, which use water as a coolant instead. Molten salt reactors can operate at higher temperatures than light water reactors, making them more efficient at generating energy. Additionally, they don’t require the high-pressure environment needed in pressurized light water reactors to prevent water from boiling, making them safer as well.
“There's a lot of work that needs to be done,” Liu said. “We need to demonstrate that the reactor is safe, and we need to demonstrate that the reactor is efficient and profitable if we build a commercial-scale reactor for generating power.”
Liu’s project, “Integrating Thermal Hydraulic Simulation and Experimentation with Data-Driven Methods to Support Molten Salt Reactors Development,” intends to streamline the development of these reactors. Specifically, ML would help researchers create better simulations and design better experiments.
One part of the proposed project focuses on physics-informed modeling, where data is collected from experiments and used to train a machine-learning model. Then, researchers add physical constraints that are consistent with reality to make sure the model matches real-world physics of a nuclear reactor.
“In this way, we ensure that the modeling tool we got is accurate and efficient and consistent with the physical constraints of the system,” Liu said.
When we develop a reactor, it is still humans making decisions. Humans need to gain insights from that big data. We need new tools to help us process that information and help us make decisions.
The project also involves using ML in the experiments themselves. Liu will use a “digital twin,” or a virtual replica of an otherwise expensive experimental facility. Not only are physical experiments expensive, but it can also be difficult to measure each crucial part of the system.
“It’s not feasible to measure all the parameters of a facility directly due to the high costs and the difficulty of accessing certain locations or measuring specific parameters,” Liu said.
To address this challenge, Liu proposes combining the physical measurements from the facility with data from a digital twin, a virtual replica of the experimental setup. This approach allows researchers to estimate the parameters that are difficult or impossible to measure directly.
“By integrating the physical measurements with the digital twin’s data, we can obtain a more comprehensive and accurate picture of the experimental results,” Liu said.
Finally, this research project will combine these two components — use of ML in simulations and experiments — to reinforce each other.
“The experiments’ measurements will inform simulations to give the model a better accuracy, while the simulation results will guide experiments to generate new, high-value data to improve the model itself,” Liu said. “It is a two-way coupling of the process.”
Liu will use this approach to investigate molten salt reactors, but he expects the idea could be applied to other kinds of nuclear reactors as well.
Analyzing such data from experiments is important for making the right decisions when designing and building new reactors. Use of computers, such as ML techniques, can support this decision-making by providing insights to the humans making the choices. This can help make advanced, innovative reactors more safe, optimized, and affordable to operate and maintain, Liu said.
“When we develop a reactor, it is still humans making decisions,” Liu said. “Humans need to gain insights from that big data. We need new tools to help us process that information and help us make decisions.”
To make this proposal a reality, Liu will work with the Center for Advanced Small Modular and Micro Nuclear Reactors (CASMR), a research center directed by nuclear engineering professor Yassin Hassan, which Liu is also affiliated with.
“We hope to have an integrated framework to demonstrate that this approach is workable and has the potential to be scaled up to a real large-scale reactor system, and to demonstrate that AI and ML can make a real impact on the nuclear engineering community,” he said.